- Home»
- Resources»
- Blog Posts»
- The Fundamentals of Artificial Intelligence (AI) and Revenue Cycle Management (RCM): A Primer
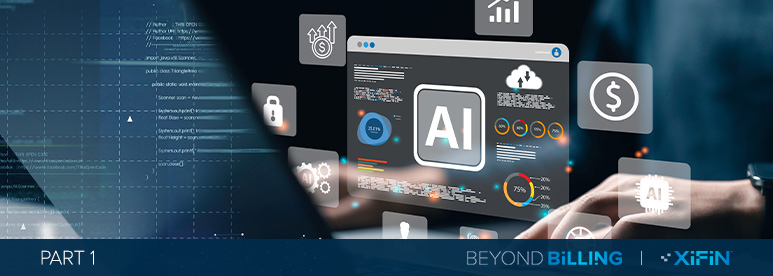
The Fundamentals of Artificial Intelligence (AI) and Revenue Cycle Management (RCM): A Primer
November 3, 2023Understanding how to apply artificial intelligence (AI) to solving real-world healthcare and diagnostics revenue cycle management (RCM) challenges requires a grounding in some fundamental definitions. This is the first in a series of posts addressing how AI is shaping RCM, and in it we’ll explore the terms and technology that form the foundation of this understanding.
When it comes to definitions and terms surrounding AI and the revenue cycle, there are four important concepts to discuss first:
Robotic Process Automation (RPA)
While RPA is not artificial intelligence, it’s important to address because it’s an approach that many organizations take to automate repetitive tasks in the RCM process before implementing AI.
RPA is a rule-based software engine. It has no intelligence; it simply automates repetitive tasks. It has a software robot that mimics human actions, and it is used to replace human keystrokes. RPA uses application programming interface (API) calls and/or automated keystroke technology to replace the activity of a human with a scripted or rules-based approach.
RPA can be used in a lot of different places when an organization is trying to automate a process. It can be combined with AI to enable automated workflows.
Machine Learning
At its most basic level, AI is concerned with the simulation of human intelligence by machines. The first AI term that is relevant to RCM is machine learning, which refers to the ability to generate a prediction; to predict an outcome by training a model on relevant historical input data.
For example, an organization may want to predict the probability of reimbursement for a particular procedure. Machine learning is a great tool to make that happen. It can study the results of historic reimbursements to determine how similar claims were paid or not paid in the past and generate a prediction along with the confidence factor to help understand the likelihood of future claims being reimbursed. At XiFin, we use machine learning a lot for these types of predictive analytics to help us understand likely outcomes.
Natural Language Processing (NLP)
Natural language processing is a very different type of AI than machine learning. It’s used to understand unstructured data, such as natural language text, and convert it into structured data that can be used in processing or as input into machine learning algorithms to create predictions.
Historically, natural language processing has been used in healthcare for clinical activities, such as tapping into unstructured clinical notes to aid in diagnosis. NLP is also used to understand and extract relevant activities and events from clinical notes to be used in structured reporting or as input into training an AI model or executing an AI-powered workflow.
Generative AI
Lastly, and where most of the AI hype is seen this year is in generative AI. Generative AI is a technology that enables a natural language processing application to not only consume natural language data but also generate relevant content in response to a prompt. ChatGPT is a great example of generative AI.
Generative AI is a powerful tool that can absorb a lot of content and a lot of information and then reorganize and structure that content and summarize it in various ways in natural language or in computing languages.
What Organizations Can Do With AI in RCM
AI can play a number of important roles in business. When it comes to RCM for laboratories and other diagnostic organizations, AI can:
Inform decision-making processes by generating new or derived data, which can then be used to take action inside a process or in reporting and analytics. Machine learning, in particular, is very powerful when it comes to informing processes by creating or deriving relevant data. It can help understand likely outcomes based on the data that is in a system. For example, machine learning can help organizations understand likely outcomes based on historical data, such as an organization’s current outstanding accounts receivables (AR), and what’s likely to happen with that AR based on historic performance.
Accelerate the creation of configurations and workflows. For example, generated or derived data can be used to create configurations within a revenue cycle workflow to address changes or shifts in likely outcomes, such as denial rates. If an organization is using AI to analyze historical denial data and predict denial rates, changes in those predicted denial rates can be used to modify a workflow to prevent those denials upfront or to automate appeals on the backend. This helps organizations adapt to changes more quickly and accelerates the time to reimbursement.
Automate workflows by providing or informing decisions directly. For example, when the AI sees shifts or changes, it knows what to do to address them. This enables an organization to take a process in the revenue cycle workflow that is very human-oriented and fully automate it.
Validate data and identify outcomes that are anomalous or that lie outside of the norm. This helps an organization ensure that the results achieved meet the expected performance, understand whether the appropriate configurations are in place, and identify if an investigation is required to uncover the reason behind any anomalies, so they can be addressed.
Generate content, such as letters or customer support materials.
In our next blog of this series, we’ll dive more deeply into the data maturity journey and its influence on effective AI adoption. In the meantime, watch a recording of our recent webinar with Dark Daily, “Unlocking RCM Potential: How AI Is Shaping Revenue Cycle Management Workflow.”