- Home»
- Resources»
- Blog Posts»
- Using Machine Learning to Improve Revenue Cycle Management (RCM) Operations and Decision-Making
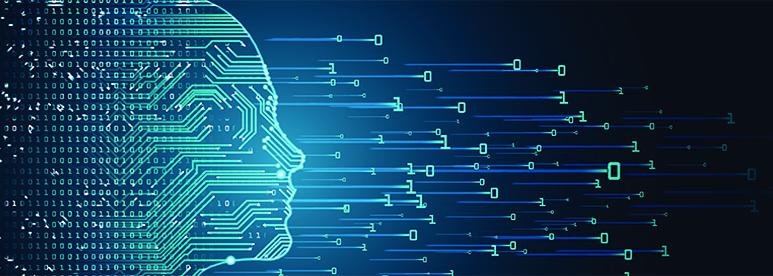
Using Machine Learning to Improve Revenue Cycle Management (RCM) Operations and Decision-Making
December 11, 2018Machine learning and artificial intelligence (AI) are mainstream topics in the technology space, but separating the truth from the hype can be a challenge. AI refers to any technique that enables computers to mimic human intelligence. Machine learning (ML) is a subset of AI and is the science of getting computers to act without having to be explicitly programmed. It does this by analyzing data and discovering patterns.
There are many AI or ML applications that can be applied in powerful ways to create insight and actions in revenue cycle management. To truly unlock that value, it’s important to have a technology partner that understands your data, your workflows, and the data science techniques to know when and how to apply the right methodology to the right problems to optimize your results. At XiFin we approached these new techniques in a thoughtful and practical way. As part of our research, we determined that the business intelligence capability of our product portfolio was the area most ripe for machine learning since it would inform other ML or AI applications within the workflow logic and claim and billing process.
Machine learning also plays an important role in business process automation. According to the McKinsey and Company article, “The Automation Imperative” published in September 2018, surveyed organizations that reported successful business process automation initiatives were “more than twice as likely as others” to state that their organizations are deploying machine learning as part of their process automation efforts.1
McKinsey and Company also surveyed life insurance and property and casualty carriers and found that 80 percent of them are not delivering high impact analytics,2 meaning that the work these firms were doing in applying ML and AI were not producing measurable improvements in business results. The main reason cited for this failure was an inability to integrate analytics into workflows and frontline systems. What’s important about this study is it reinforces that high impact analytics are those that impact decisions made in the workflow, on the front-line.
At XiFin, we can apply machine learning to your clinical, diagnostic and financial data to help you:
We make these new insights available to you through visualization tools and business intelligence analytics. These insights are then used to drive process improvements. One of the most important aspects of machine learning is the feedback loop into the business process. In revenue cycle management (RCM), this typically takes the form of integrating the machine learning feedback back into the RCM workflow processes.
McKinsey and Company also published an infographic on the topic of “Using machine learning to unlock value across the healthcare value chain.” One of the use cases for machine learning applications in healthcare highlighted in this infographic is improving “claim accuracy” and reducing “administrative inefficiencies (e.g., during claims submission and processing).” 3 This use case goes on to show how machine learning was used to “identify claim recovery cases for unbundling of services.”
Another place where RCM technology companies have explored using machine learning is in forecasting expect pricing, i.e. how much a lab can expect to be reimbursed for a particular claim by the payor. At XiFin, we have found that the understanding, quality, and organization of your data in our RCM product, RPM 10 is such that a predictive analytic model works exceedingly well in predicting expect pricing. There are just a few specific inputs, which when correctly applied, can provide a highly accurate prediction. With this deep knowledge of the data, we are able to give our clients the ability to automate expect price analysis and enable better accuracy of expect pricing. As a result of these analytics, XiFin RPM will further assist our clients’ finance team better forecast reimbursements.
Here at XiFin, we are proactively working on the next best applications for machine learning and predictive analytics to advance your business. Potential new applications for machine learning include:
Unlike the insurance carriers surveyed in the McKinsey study, XiFin is using machine learning to tackle specific needs to close performance gaps and improve operational efficiency and effectiveness. We’ll continue to apply advanced analytics and machine learning where it can positively impact clinical and operational performance.
To learn more visit:
- McKinsey and Company, “The Automation Imperative,” September 2018
- McKinsey and Company, “Raising Returns on Analytics Investments in Insurance,” July 2017
- McKinsey and Company, “Using machine learning to unlock value across the healthcare value chain,” July 2018
- XiFin, for more information about the capabilities of machine learning and XiFin RPM